Research
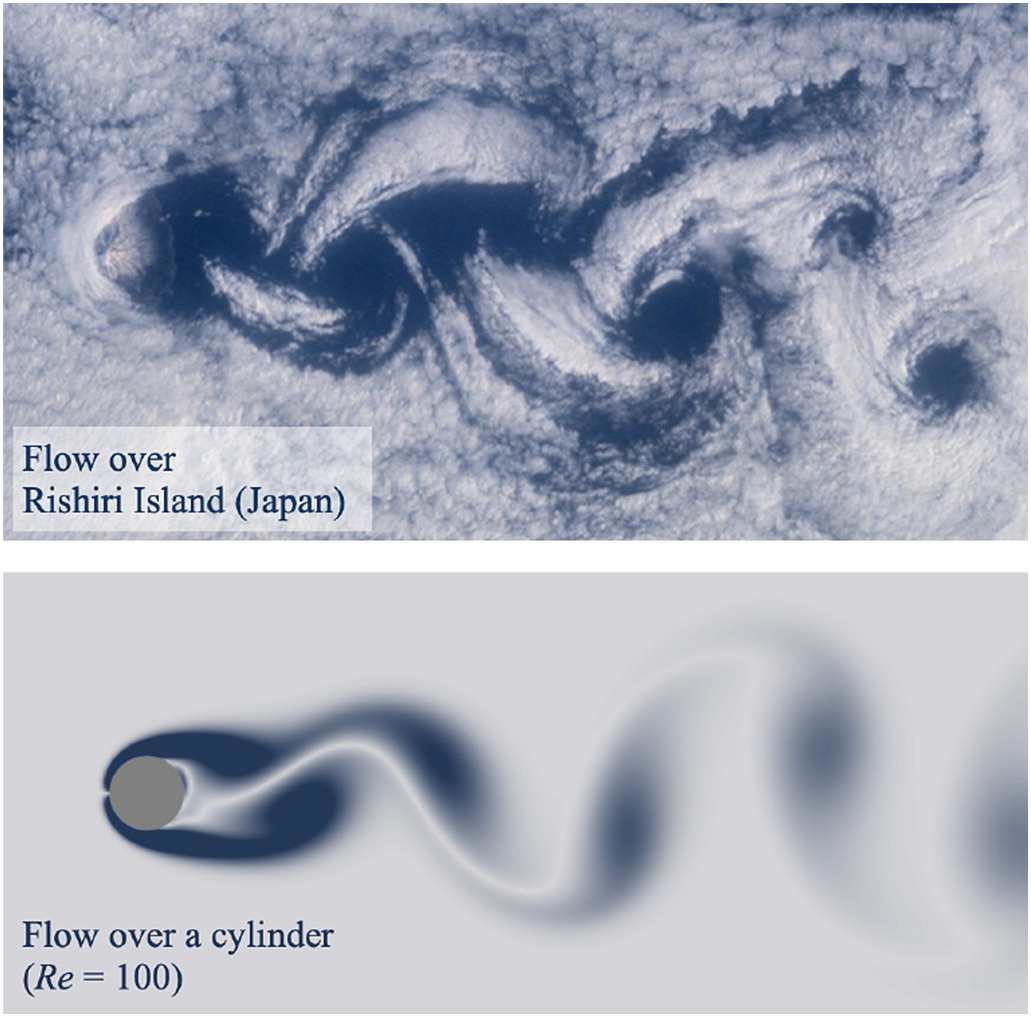
Deterministic Laws with Data-Driven Insights to Improve Fluid Predictions for Complex Systems
Fluids in motion exhibit complex phenomena that are challenging to predict but are crucial in technology, engineering, and science, including renewable energy and weather forecasting. The figure above compares the Von Karman vortices observed in the flow over Rishiri Island with a numerical simulation of flow around a 2D cylinder.I develop accurate and efficient numerical algorithms to extend the predictability horizon for the simulation of turbulent flows by addressing data uncertainty and the chaotic behavior of flows. Advancements in these methods are essential for studying climate patterns, energy production, and global warming.
My research interests include:
- Data assimilation
- Machine learning, Deep learning, Probabilistic graphical models, Deep reinforcement learning
- Numerical analysis, Scientific computing
- Computational fluid dynamics
- Finite element methods
- Ensemble simulations
- Turbulence modeling
I have authored 7 peer-reviewed publications including 3 solo papers, with 2 are currently under review on topics including the Navier-Stokes equations (NSE), adaptive penalty methods, ensemble simulations, data assimilation with nudging, Unsteady Reynolds-Averaged Navier-Stokes (URANS) models, and deep learning applications.
I am currently working on two projects:
1. Data assimilation for correcting model errors, which will soon appear on arXiv.
2. Analyzing the uniqueness of solutions for the 1-equation turbulence model of Prandtl and Kolmogorov.
Additionally, I have delivered 5 conference talks in the United States and internationally.
ResearchGate: ResearchGate Profile
Google Scholar: Rui's Google Scholar Page